For plastics manufacturers and the companies that make the industry’s machinery, AI promises to provide predictive power, higher efficiency and competitive advantage. With all the hype around AI, C-suites are under a great deal of pressure to ensure their organizations have deployed it, and let’s be honest — the hype is justified. AI is providing those companies who have successfully harnessed its power significant competitive advantage.
But AI is not a set-it-and-forget-it kind of technology. Far too many companies treat AI like a shiny new toy they deploy without putting enough thought into their goals for it or how they can ensure success. Achieving real value from AI requires a focused, comprehensive strategy with specific, tangible business goals as its aim.
Does the organization want AI to power predictive maintenance for factory machinery to lengthen its life and reduce downtime? Does management need better visibility into the supply chain or AI’s help in making a multi-sourcing strategy possible so the organization can increase its resilience in the face of supply chain volatility? Or perhaps the most pressing need is producing accurate demand forecasts to lower the risks of excess or insufficient inventory?
An AI project is a transformative project, and organizations can dramatically increase the likelihood of success by first determining where this technology could have the most impact, and then developing a strategy to achieve the desired outcome. Success with AI requires support from four fundamental pillars: the right people, the right processes, the right systems and — an element that many overlook — the right data.
The right people
AI success requires specialized skills, starting with a data analyst or data scientist. AI doesn’t produce its insights in a vacuum. It needs a lot of relevant data, formatted properly so the AI can learn and, eventually, produce accurate results and insights. Also, AI requires close management to track for biases, known and unknown, that could, over time, produce inaccurate insights.
The AI project team will need a technology adviser who can guide choosing, deploying, integrating and maintaining the hardware and technology stack required to run the AI — it’s a processing-intensive technology, and frequently needs high-performance machines to process massive amounts of data fast enough to produce timely insights. Additionally, the data will need to be stored in a scalable infrastructure with a network that can deliver it rapidly wherever it’s needed.
Collaboration between the technical and business teams is vital. Business owners must be part of the process early on to make sure that the project will truly support business goals.
The right processes
AI can produce incredible insights, but if they aren’t actionable, what’s the point? Organizations must lay out the steps people will follow to achieve the desired business objectives and then note where AI is best able to help achieve them. Also, remember that people are not gears in a machine — they need to know both what they’re expected to do and why what they’re doing is important. Without that understanding, people may drop key parts of the process because they simply don’t understand how vital they are to the outcome.
Build in measurements and feedback loops so you can gauge how well the project is progressing towards its goals. Continuous improvement is a watchword in our industry, and it applies to IT just as much as it does to manufacturing. But continuous improvement must be done in a cohesive manner that enables meeting business objectives.
The right systems
AI’s technical requirements can be high. If the use case demands it, IT may need to deploy high-performance computing (HPC) clusters powered by graphical processing units (GPUs). The cloud or even a mix of cloud and on-premises deployments are possibilities, but in some cases, organizations will need to deploy their AI on-premises.
Building the supporting infrastructure is a complex task that requires the right people with the right skills, because it's easy to pay too much and deploy more supporting technology than is necessary for an AI deployment. On the other hand, without the right underlying systems, the AI won’t be able to grow or provide insights within a timeframe that will enable the business to act on them.
It's time-consuming, expensive and complex to train a generic AI to a specific purpose, and manufacturers can reduce risk by choosing an AI platform that’s pre-trained and specifically developed for the desired use case. Additionally, this enables the manufacturer to rely on the AI vendor’s expertise and counsel about the underlying resources it will require.
The delivery of the data to decision makers is an often-forgotten element in the system pilar. Less is often more in data delivery.
The right data
AI requires a ton of data, but in addition to volume, it needs the right kind of data: specifically, a variety of data types to increase accuracy. Manufacturers should digitize internal information to the highest degree possible. HR information, purchase orders, shipping labels — AI can’t use this data if it’s stored in a file cabinet. Reliable data that allows for measuring and actioning against organizational objectives are critical — the wrong data introduces noise that can obscure insights or, worse, produce inaccurate predictions.
IoT is a useful technology for collecting relevant data: machine performance, temperature, asset movement, inventory — this and much more can be captured digitally with the right IoT solution. The more relevant data here is, the more value a manufacturer can extract from it.
External data sources can also enrich and increase the value of internal data. Weather patterns, macro- and micro-economic data, highway traffic patterns — depending on the use cases, it can all increase the accuracy of AI’s predictions. Data must be intelligent and actionable, not just informational.
“It’s a journey, not a destination” is a business cliché, but in the case of AI, the cliché fits because it’s true. Ensuring the fundamentals — People, Processes, Systems and Data — are all in place for an AI project vastly increases the chance of success.
Gavin Verreyne is Senior Vice President Client Success and Digital Transformation at Syspro, which produces enterprise resource planning software for industries in the manufacturing and distribution sectors.
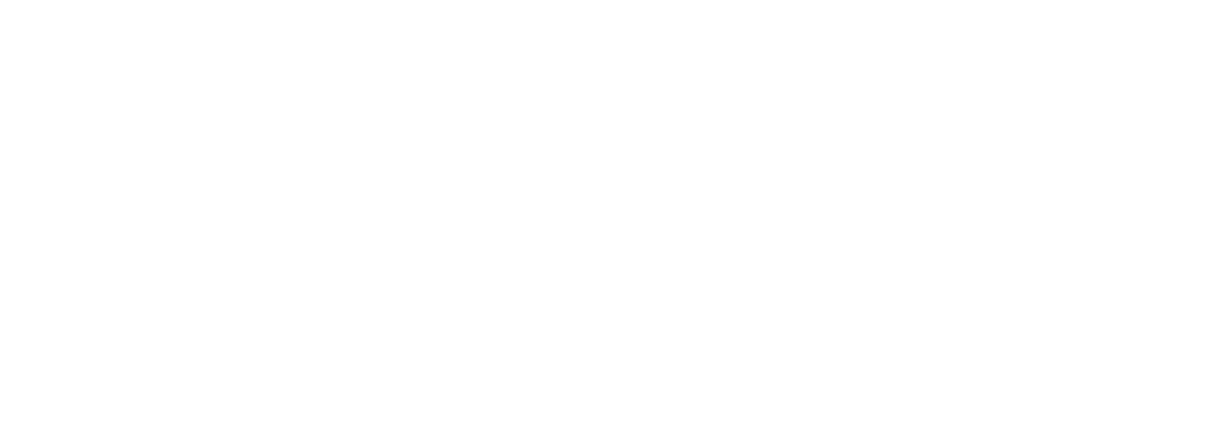